Overview
Quantum Machine Learning (QML) is considered to be a promising early quantum computing application in the NISQ era. The Variational Quantum Algorithm (VQA), one of the near-term QML architectures, uses an ansatz to learn a quantum state, created using unitaties with tunable parameters. This architecture allows us to construct a shallow circuit suitable for NISQ devices. Embedding an input classical data into a Hilbert space prior to the ansatz (so-called feature map), the VQA provides a means of analyzing the classical data for classification, regression, optimization and so on.
Research
The early studies with VQA include the classification of a physics process predicted in Supersymmetry from a Standard Model background process, showing that the QML has a comparable performance to the classical ML approaches when the sample size is modest. We will further explore the VQA approach for applications in high-energy physics and industrial problems, with research interests on the optimization in data embedding, learning architecture, gradient-based parameter tuning and so-called “barren plateau” problem (vanishing gradient with the number of qubits).
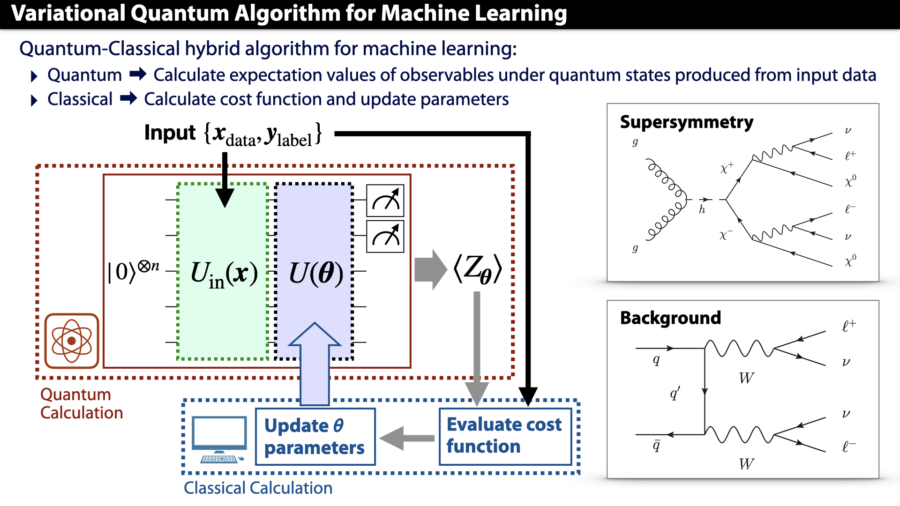